The enigmatic Channel State Information (CSI) is an essential ingredient in MIMO systems that enables the transmission of high-quality signals. CSI provides a window into the channel’s elusive characteristics, such as fading and interference, which are crucial for optimizing signal transmission. With MIMO systems relying on multiple antennas to transmit and receive data simultaneously, accurate CSI becomes indispensable for efficient communication.
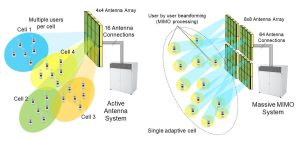
Compression techniques for CSI take center stage in reducing feedback overhead without sacrificing reconstructed CSI quality. Lossy compression methods discard some information during compression to minimize feedback overhead. Conversely, lossless compression methods retain all information but require more bandwidth for feedback. Therefore, selecting the most appropriate compression method hinges on several factors like available bandwidth and reconstruction accuracy requirements.
Deep learning-based approaches have emerged as promising solutions to enhance the efficiency of CSI compression by reducing feedback overhead while maintaining high reconstruction quality. Deep convolutional neural networks prove useful in massive MIMO system csi-feedback due to their ability to unravel complex patterns in data better than traditional linear models can muster. These deep learning-based methods also provide answers to challenges associated with limited feedback channels and delay issues commonly encountered in massive MIMO systems.
In summary, having precise Channel State Information remains paramount when it comes down optimizing signal transmission within MIMO systems. Compression techniques serve as critical ingredients towards minimizing feedback overhead without compromising reconstructed accuracy or quality one bit! With deep learning-based approaches providing promising solutions addressing massive MIMO system csi-feedback challenges while retaining high-quality reconstructed CSI necessary for effective communication between devices or networks – there can be no doubt about their usefulness!
Compression Techniques for CSI: Explore the different compression techniques available for CSI, including lossy and lossless methods, and their impact on the quality of the reconstructed CSI.
Contents
- 1 Compression Techniques for CSI: Explore the different compression techniques available for CSI, including lossy and lossless methods, and their impact on the quality of the reconstructed CSI.
- 2 Deep Learning and CSI Compression: Discover how deep learning can be used in CSI compression to improve the compression ratio and reduce feedback overhead.
- 3 Massive MIMO Systems and CSI Feedback: Understand the challenges associated with CSI feedback in massive MIMO systems, including limited feedback and feedback delay, and explore the methods used to address them.
- 4 Compression for Massive MIMO Systems: Learn about the different compression strategies used in massive MIMO systems and their impact on the quality of the reconstructed CSI.
- 5 Neural Networks for CSI Compression: Explore how neural networks can be used for CSI compression and feedback, including autoencoder-based approaches and deep learning-based methods.
- 6 FDD Massive MIMO System and Channel Estimation: Understand the challenges associated with channel estimation in FDD massive MIMO systems and the methods used to overcome them.
- 7 Practical Considerations for CSI Compression: Learn about the practical considerations for CSI compression in MIMO systems, including the impact of feedback overhead and the trade-offs between compression ratio and reconstruction quality.
The realm of CSI compression is rife with a myriad of techniques, ranging from lossy to lossless methods. The former is widely utilized in MIMO systems as it effectively reduces feedback overhead while still maintaining an acceptable level of reconstruction quality. The key lies in discarding non-critical information that isn’t pertinent for signal transmission. Conversely, the latter aims to preserve every bit and byte without any compromise on quality.
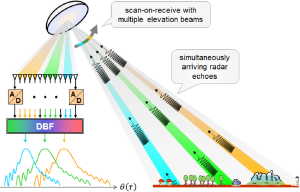
Given the gargantuan number of antennas present in massive MIMO systems, convolutional compression reigns supreme due to its unparalleled ability to handle enormous datasets efficiently. This technique processes data through a sequence of filters that extract relevant features before compressing them further. But compressing CSI for these behemoth systems must be approached cautiously since accuracy in channel estimation plays an indispensable role in signal transmission.
Enter deep learning – a promising approach that has taken the world by storm with its ability to improve CSI compression and feedback performance significantly. Neural networks have the uncanny knack for picking up complex patterns from vast amounts of data and leveraging this knowledge to generate compact representations with minimal distortion effortlessly. Autoencoder-based approaches alongside other deep learning-based methods are just some examples of neural network-based techniques that have demonstrated significant improvements in reducing feedback overhead whilst simultaneously preserving high-quality reconstructed CSI within MIMO systems.
Deep Learning and CSI Compression: Discover how deep learning can be used in CSI compression to improve the compression ratio and reduce feedback overhead.
The use of deep learning in compressing CSI data for MIMO systems has caused quite a stir in the technological world. The neural networks employed to achieve this feat are nothing short of remarkable, boasting impressive compression ratios and reduced feedback overhead that prove particularly useful in massive MIMO channels. Through training these deep neural networks on vast sets of channel data, one can unearth underlying patterns and correlations that exist between different aspects of the channel – a true marvel indeed.
However, there is no denying that using deep learning for massive MIMO CSI compression poses its fair share of challenges. One such challenge is accurately estimating the channel – an issue amplified by interference from neighboring cells and other factors in FDD massive MIMO systems. Despite this obstacle, recent research indicates that combining advanced estimation methods with deep learning-based compression techniques can result in high-quality downlink CSI reconstruction even amidst complex environments.
Another vital aspect to consider when utilizing deep learning for massive MIMO CSI compression is selecting an appropriate feedback scheme. With varying schemes come differing trade-offs between feedback overhead and compression rate; it’s essential to choose one tailored to your specific needs while still maintaining acceptable levels of accuracy when reconstructing the CSI data. All things considered, though, exciting developments leveraging deep learning techniques promise drastic improvements both efficiently and effectively within massive MIMO systems!
Massive MIMO Systems and CSI Feedback: Understand the challenges associated with CSI feedback in massive MIMO systems, including limited feedback and feedback delay, and explore the methods used to address them.
The issue of limited feedback is a perplexing challenge in massive MIMO systems. With an overwhelming number of antennas and users, transmitting complete CSI matrices for each user is simply impractical. As a result, there exists a trade-off between the quantity of feedback overhead and the quality of CSI estimation. In order to surmount this hurdle, machine learning techniques have been implemented to compress the CSI information while still maintaining its precision.
DL-based CSI compression has burst onto the scene as an effective solution for curtailing feedback overhead. By exploiting spatial correlations among different antennas and users, deep convolutional compression for massive MIMO csi can attain high compression ratios with low distortion. Furthermore, these methods utilize bi-directional channel reciprocity in deep learning to refine channel estimation and feedback.
Another approach to resolving limited feedback involves adaptive compression strategies that adjust their learning rate based on available bandwidth or signal-to-noise ratio (SNR). For instance, convolutional compression for massive MIMO systems can adaptively compress the CSI information depending on SNR levels without compromising reconstruction quality. Such methodologies optimize resource utilization by only compressing what’s essential whilst preserving high-quality channel estimates for optimal system performance.
All in all, myriad methods exist to tackle limited feedback associated with massive MIMO systems such as DL-based CSI Compression or Adaptive Compression Strategies etc., necessitating appropriate method selection according to specific needs considering factors like Feedback Overhead etc.. The continued development of new technologies will further improve these approaches leading towards better communication networks in future applications; surely something worth bursting about!
Compression for Massive MIMO Systems: Learn about the different compression strategies used in massive MIMO systems and their impact on the quality of the reconstructed CSI.
The limited feedback capacity in massive MIMO systems presents a significant challenge, requiring the compression of CSI before it’s sent back to the base station. Commonly used lossy compression techniques achieve higher ratios than their lossless counterparts, but what is their impact on reconstructed CSI quality? It must be evaluated with care.
Luckily, deep learning-based CSI compression and feedback approaches show promise when tackling low rate massive MIMO CSI feedback. These methods leverage neural networks to learn a mapping between compressed and uncompressed CSI, leading to better reconstruction quality while reducing feedback overhead. It’s been successful in multi-user massive MIMO scenarios where each user’s channel information can be effectively compressed and transmitted.
However, for FDD massive MIMO systems, channel estimation becomes even more challenging due to inter-cell interference and frequency-selective fading. Pilot contamination mitigation techniques that exploit spatial correlation across antennas or time-frequency resources are often employed in practical MIMO designs to overcome these issues. By combining these strategies with efficient CSI compression approaches like deep learning-based ones for time-varying massive MIMO channels – we can recover accurate channel information at lower rates without sacrificing system performance.
Neural Networks for CSI Compression: Explore how neural networks can be used for CSI compression and feedback, including autoencoder-based approaches and deep learning-based methods.
The potential of neural networks in reducing CSI feedback overhead and improving compression ratio is undeniable. However, when dealing with time-varying massive MIMO channels where the channel state information changes rapidly, traditional techniques may not suffice. Fear not though! Deep learning-based methods can adapt to these changes and provide accurate reconstruction of the original CSI matrix.
Autoencoder-based approaches have gained popularity recently as one type of deep learning-based channel estimation and feedback technique. By utilizing a neural network architecture consisting of an encoder and decoder, they compress original CSI values into a lower-dimensional representation whilst preserving important features. This compressed data is then transmitted through the uplink for further processing at the receiver end – pretty nifty!
Another approach involves using neural networks for CSI feedback by learning feedback weights from historical data. This method takes advantage of nearby users’ csi values to improve accuracy by leveraging correlations between them; talk about smart! Additionally, it reduces computational complexity compared to other compression techniques found in literature.
In massive MIMO scenarios with many antennas, conventional methods can lead to high overheads due to large amounts of data transmission required for each antenna element’s csi value; yikes! Not all hope is lost though – deep learning-based methods offer an efficient alternative that reduces this overhead while still maintaining good quality reconstructed csi matrices on both ends of communication links.
FDD Massive MIMO System and Channel Estimation: Understand the challenges associated with channel estimation in FDD massive MIMO systems and the methods used to overcome them.
The task of channel estimation in FDD massive MIMO systems poses a formidable challenge, fraught with feedback errors and delay. These hindrances can wreak havoc on the accuracy of CSI feedback, leading to a nosedive in system performance. Multiple-rate compressive sensing has emerged as a potential solution for this conundrum, allowing for efficient compression of CSI data while curbing the impact of feedback errors.
Another approach that holds significant promise is deep learning-based methods. By delving into neural networks to unravel patterns within real-life CSI data, these techniques have demonstrated their prowess in enhancing compression ratios and minimizing overheads while maintaining high reconstruction quality. They can even predict missing information from incomplete or noisy measurements with remarkable accuracy.
Aside from channel estimation and CSI compression lies another critical obstacle – effective feedback management in FDD multi-user massive MIMO systems. One possible fix involves channelling predictions based on past observations and statistical models, facilitating accurate forecasts of future channel conditions despite limited current information without putting undue stress on computation resources or bandwidth usage for continuous updates.
Overall, these cutting-edge methods offer tremendous potential towards achieving reliable communication across vast wireless networks by effectively mitigating some significant challenges associated with Channel Estimation and Feedback Management processes within FDD Massive MIMO Systems.
Practical Considerations for CSI Compression: Learn about the practical considerations for CSI compression in MIMO systems, including the impact of feedback overhead and the trade-offs between compression ratio and reconstruction quality.
The implementation of CSI compression in practical MIMO settings begs the question: what about feedback overhead? As compressed CSI data increases, so does the need for accurate transmission feedback. This can cause delays and a decrease in system efficiency. However, fret not! Reduced-feedback methods like quantization-based techniques or matrix compression can be implemented to address this issue.
Traditional CSI generation is done through pilot signals transmitted by the transmitter and received by the receiver. But there’s a catch – noise or interference may lead to inaccurate estimates. To combat this, additional compression techniques can be used with traditional estimation methods to improve accuracy.
In massive MIMO systems using hundreds or thousands of antennas, DL-based CSI feedback has proved groundbreaking over traditional approaches. By adopting neural networks for compressing and reconstructing CSI data, higher ratios of compression are achievable without compromising quality significantly. Not only that; these methods also reduce overall feedback overhead while enhancing system performance during real-world tests!