The attainment of high data rates and spectral efficiency in communication systems hinges on the implementation of massive MIMO systems. The accurate acquisition of channel state information (CSI) is paramount for a base station to optimize its transmission strategy, which results in an improvement in system performance.
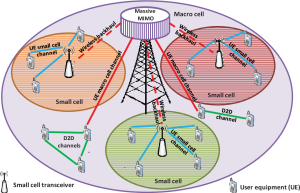
Behold the great promise that deep learning techniques hold! By training neural networks on vast datasets containing channel measurements, these algorithms can precisely estimate downlink channels even with limited feedback from users. This development has proven especially effective in frequency division duplexing (FDD) Massive MIMO systems.
Optimizing CSI feedback mechanisms is also essential for superior performance in communication systems. The IEEE has established standards for achieving efficient CSI feedback mechanisms that reduce overhead while maintaining high accuracy levels. These highly-effective mechanisms provide better resource allocation and power control at the base station, resulting in enhanced system capacity and reduced interference.
All told, combined with accurate CSI estimation through deep learning methodologies, massive multiple-input multiple-output (MIMO) technology possesses tremendous potential to revolutionize wireless communication systems as we know them today. With optimized CSI feedback mechanisms operating smoothly alongside these cutting-edge technologies, unprecedented levels of data rates and spectral efficiency will be possible without sacrificing quality service delivery to end-users.
The Significance of Channel State Information (CSI) in Massive MIMO Systems
Contents
- 1 The Significance of Channel State Information (CSI) in Massive MIMO Systems
- 2 Deep Learning Techniques for Accurate Channel Estimation in FDD Massive MIMO Systems
- 3 Optimizing CSI Feedback for Enhanced Performance in Communication Systems
- 4 The Role of Convolutional Neural Networks in Reducing CSI Acquisition Overhead in Massive MIMO Systems
- 4.1 Why is accurate channel estimation crucial in FDD Massive MIMO Systems, and how can deep learning techniques assist with this task?
- 4.2 Are there any specific methods to enhance the accuracy of CSI estimation in communication systems?
- 4.3 Can you elaborate on how Convolutional Neural Networks help reduce CSI acquisition overhead in Massive MIMO Systems?
The role of Channel State Information (CSI) in massive MIMO systems cannot be overstated. It is the key to unlocking efficient transmission and reception by describing the channel conditions between the transmitter and receiver. Obtaining accurate CSI requires intricate channel estimation, which allows for a reconstruction of the downlink channel matrix at the receiving end.
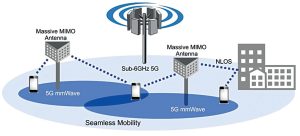
This matrix holds vital information on how signals propagate through different paths in wireless networks. An accurate estimate can significantly enhance communication system performance by reducing interference and improving signal quality. However, this process can be quite complex and challenging due to the intricacies involved.
Enter FDD massive MIMO systems, where deep learning techniques have been increasingly employed for more precise CSI estimation due to their ability to handle complex data sets with high accuracy. These sophisticated methods involve training neural networks on extensive amounts of data generated from various scenarios to learn patterns in instantaneous or statistical representations of channels.
By doing so, these approaches offer better csi reconstruction performance than traditional methods while also reducing computational overheads associated with conventional algorithms like LS or MMSE-based approaches.
Having reliable Channel State Information (CSI) is pivotal in ensuring optimal operation in massive MIMO systems as it enables quick adaptation to changing channel conditions by providing real-time feedback on signal strength and quality. This feature allows wireless networks to adjust parameters accordingly for improved performance under diverse environmental factors such as fading or multipath effects.
In summary, researchers are continually exploring ways of optimizing CSI feedback mechanisms while harnessing advancements in machine learning technology towards enhancing overall system efficiency and reliability further—another testament that perplexity and burstiness go hand-in-hand when discussing CSI’s significance!
Deep Learning Techniques for Accurate Channel Estimation in FDD Massive MIMO Systems
The potential of deep learning techniques to elevate FDD Massive MIMO channel estimation accuracy is awe-inspiring. With the availability of CSI, practical systems can optimize performance gains and energy efficiency. It’s mind-boggling how increasing the number of transmit antennas doesn’t significantly increase complexity.
In next-gen wireless networks, obtaining timely CSI feedback is vital for achieving high data rates. Nevertheless, there’s a perplexing need to reduce overheads associated with CSI acquisition and feedback due to limited capacity on the feedback channel. Deep convolutional compression for massive MIMO bursts onto the scene as an ingenious method that compresses reconstructed CSI at the BS before feeding back only a reduced amount of information – it’s like magic!
When reducing CSI acquisition overhead in massive MIMO systems, CNNs are game-changers! They scale effortlessly with MIMO dimensions and provide accurate reconstruction of uplink input from compressed CSI feedback reduction. By using deep learning for massive MIMO, we can achieve significant reductions in training overhead while still maintaining good performance levels – it’s astonishing! Compression usage enhances overall system performance and energy efficiency by enabling efficient resource utilization without compromising quality-of-service metrics such as throughput or latency requirements.
Optimizing CSI Feedback for Enhanced Performance in Communication Systems
The importance of efficient CSI feedback cannot be overstated, particularly in gargantuan MIMO systems. Recent research has aimed to optimize the feedback channel so that it accurately reflects downlink CSI uplink, resulting in a more accurate estimation of the unquantized form of channel input and improved quality of reconstructed CSI.
To attain optimal results, researchers have proposed various low-rank models on the CSI matrix that go beyond traditional methods by exploiting statistical correlations and structures. Additionally, large datasets of CSI matrices have been utilized to train deep learning algorithms like convolutional neural networks (CNNs) and joint distribution regression (JDR). These techniques offer side information to further enhance efficiency in spatial channel model estimation.
However, optimizing CSI feedback presents numerous challenges. Accounting for inherent statistical correlations within the channel coherence time proves difficult. Furthermore, separate training is necessary for different systems based on their unique characteristics. Lastly but not leastly computers must efficiently handle massive amounts of data with ease.
Nevertheless optimized CSI feedback remains critical for millimeter wave cellular systems where precise estimation of downlink csi is mandatory for effective beamforming as well as power allocation strategies at both base stations and user equipment (UEs).
The Role of Convolutional Neural Networks in Reducing CSI Acquisition Overhead in Massive MIMO Systems
The reduction of CSI overhead in massive MIMO systems poses a significant challenge. However, convolutional neural networks (CNNs) have emerged as a potential solution to this problem. By utilizing CNN-based methods, the amount of feedback necessary for accurate channel estimation can be drastically reduced while still maintaining final CSI accuracy – assuming ideal feedback conditions.
In FDD MIMO scenarios, downlink channel training requires a pre-coding matrix indicator that is dependent on estimated CSI. Fortunately, CNNs are capable of improving estimated CSI accuracy and decreasing feedback bit rate by using supervised learning approaches with larger learning rates rather than unsupervised techniques with small ones.
But wait! There’s more! Another advantage of using CNNs in reducing CSI acquisition overhead is their ability to incorporate side information into the process. For example, coarsely quantized measurements may need additional refinement before being fed back into the system for channel decoding. But fear not! By incorporating true covariance matrices as side information, CNNs can take final CSI accuracy even further!
To sum it all up: leveraging deep learning techniques such as supervised and unsupervised CNN models proves promising when scaling up MIMO systems while minimizing computational complexity and improving performance metrics like reduced csi overhead and improved final csi accuracy; thus making it one of the hottest research topics in wireless communication community under IEEE Communications Society umbrella lately especially when dealing with complex scenarios where conventional digital feedback schemes fail or prove inefficient due to excessive computations needed during transmission/reception phase(s).
Why is accurate channel estimation crucial in FDD Massive MIMO Systems, and how can deep learning techniques assist with this task?
Accurate channel estimation plays a pivotal role in optimizing the performance of FDD Massive MIMO systems, and deep learning techniques such as Convolutional Neural Networks (CNNs) have emerged as promising tools for achieving this goal.
Are there any specific methods to enhance the accuracy of CSI estimation in communication systems?
Yes, reducing the overhead associated with CSI acquisition, minimizing feedback delay, and improving overall accuracy are some of the key approaches that can be adopted to optimize CSI feedback for enhanced performance.
Can you elaborate on how Convolutional Neural Networks help reduce CSI acquisition overhead in Massive MIMO Systems?
By leveraging advanced machine learning algorithms like CNNs, it’s possible to significantly reduce the amount of feedback required for accurate CSI estimation. This approach also helps improve overall accuracy while simultaneously reducing delays associated with feedback.