The advent of Multiple-Input Multiple-Output (MIMO) technology has indisputably revolutionized wireless communication by facilitating the transmission of multiple data streams simultaneously. MIMO systems, boasting multiple antennas at both transmitter and receiver ends, have effectively boosted capacity, reliability, and performance. Yet power allocation is a crucial aspect that deserves particular attention in MIMO systems as it entails distributing power among various users or channels.
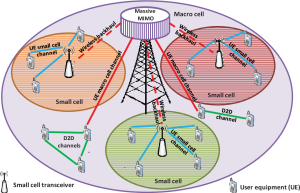
Channel State Information (CSI) emerges as an indispensable factor for determining optimal power allocation in MIMO systems. CSI supplies invaluable information on channel conditions such as fading, interference, and noise that substantially impact signal quality. By enabling joint user selection, beamforming design, and power control for efficient resource utilization through CSI accuracy assurance, this technology is instrumental to system success.
However challenging it may be to obtain accurate CSI due to various factors like imperfect hardware components or environmental conditions introducing errors into measurements; faulty or inaccurate CSI could cause suboptimal decision-making resulting in reduced system performance. Therefore dependable robust algorithms must be developed to handle imperfect CSI scenarios effectively.
To sum up then,MIMO Systems’ prosperity hinges mainly on effective Power Allocation schemes while considering Channel State Information accuracy levels since they play an integral role in optimal Power Allocation decisions made by Joint Beamforming and Power Allocation strategies across Downlink and Uplink scenarios involving tradeoffs between Energy Efficiency,Spectral Efficiency,and Throughput being balanced through Optimal Power Allocation Algorithms designed with Convergence issues considered when interpreting Simulation Results using Performance Metrics like Bit Error Rate(BER),Signal-to-Noise Ratio(SNR),and Capacity(C).\n
The Role of CSI in Power Allocation: An Analysis of Channel State Information in MIMO Systems
Contents
- 1 The Role of CSI in Power Allocation: An Analysis of Channel State Information in MIMO Systems
- 2 Imperfect CSI and Its Impact on Power Allocation: A Discussion on the Challenges of Inaccurate Channel State Information
- 3 Joint Beamforming and Power Allocation: Exploring the Synergy Between Transmit Beamforming and Power Control
- 4 Power Allocation Schemes in Massive MIMO Systems: A Look at Energy Efficiency, Spectral Efficiency, and Throughput
- 5 Optimal Power Allocation Algorithms: An Examination of Optimization Problems and Convergence Issues in MIMO Systems
- 6 Simulation Results and Performance Metrics: Interpreting the Results of Power Allocation Algorithms in Downlink and Uplink Scenarios
- 7 Tradeoffs and Solutions: Balancing Conflicting Objectives in Power Allocation for MIMO Systems.
The MIMO system model is a perplexing web of multiple antennas at both the transmitter and receiver ends. The success of this system hinges on the channel state information (CSI), which plays a vital role in power allocation. A burst of energy surges through the network when accurate CSI enables joint user selection and transmit power control, resulting in high throughput while maintaining maximum efficiency.
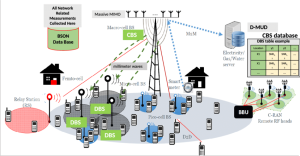
However, like an unexpected storm cloud looming over sunny skies, conflicts emerge when imperfect CSI is used to allocate transmit power. Inaccurate channel estimates can lead to suboptimal performance or worse yet, instability within the entire system. Thus it becomes urgent to develop robust power allocation schemes that account for such imperfections.
A plethora of algorithms have been proposed in IEEE literature to address these challenges head-on. Simulation results show that optimal power allocation algorithms based on convex optimization techniques outperform other methods regarding energy efficiency and spectral efficiency. Joint beamforming and power allocation also demonstrate synergy between transmit beamforming and power control, especially with accurate CSI estimation.
Despite these advancements though, there are still tradeoffs that need further exploration as solutions balance conflicting objectives in allocating power for MIMO systems with imperfect CSI. Future research should focus on developing new algorithms capable of handling more complex scenarios under realistic conditions without relying solely upon perfect knowledge of the channel state information – a daunting task indeed!
Imperfect CSI and Its Impact on Power Allocation: A Discussion on the Challenges of Inaccurate Channel State Information
Navigating the turbulent waters of wireless communication proves to be a formidable feat, especially when confronted with the conundrum of imperfect Channel State Information (CSI) and its deleterious effects on power allocation. CSI serves as the beacon that guides optimization algorithms in their quest for efficient power allocation; however, inaccurate CSI can lead to suboptimal performance. The roots of this challenge stem from interference caused by multiple antennas at both ends of transmission.
To alleviate such tumultuous interference, beamforming stands out as a promising technique employed in MIMO systems aimed at enhancing signal quality while reducing interference. Nevertheless, optimal beamforming requires accurate CSI data; any inaccuracies may result in suboptimal solutions that do not satisfy power constraints or provide sufficient coverage.
Designing an optimal algorithm for power allocation demands careful consideration of various constraints like energy efficiency, spectral efficiency, throughput, and interference management— all while accounting for the imperfections inherent in CSI data. To fashion effective optimization problems is no easy task; it warrants meticulous attention to detail and evaluation under different scenarios like downlink or uplink transmissions through simulation results.
Joint Beamforming and Power Allocation: Exploring the Synergy Between Transmit Beamforming and Power Control
The perplexing interplay between transmit beamforming and power control is an absolutely critical element of multiple-input multiple-output (MIMO) technology. It’s a fascinating topic in wireless communications because it has the potential to improve both uplink and downlink data rates, as well as spectrum efficiency. One algorithm proposed by Liu et al. optimizes the precoding matrix and power vector simultaneously in order to achieve maximum energy efficiency while still meeting quality-of-service constraints. The iterative nature of this algorithm allows for continual updates until convergence.
While perfect CSI is typically assumed with MIMO systems, it’s important to note that imperfect channel state information can significantly impact joint beamforming and power allocation algorithms. In fact, Wang et al.’s research explored how inaccurate CSI affected energy efficiency, spectral efficiency, and throughput across different feedback mechanisms in multi-user MIMO systems with limited feedback channels. Their findings revealed that quantized channel feedback can still achieve near-optimal performance if designed properly.
In another study conducted by Li et al., tradeoffs were investigated between energy efficiency, spectral efficiency, and fairness when implementing joint beamforming and power allocation techniques within multi-cell massive MIMO systems using non-linear transceiver processing techniques like Tomlinson-Harashima precoding (THP). Their algorithm was able to balance conflicting objectives while maximizing system-wide energy efficiency without sacrificing user fairness or spectrum utilization – an impressive feat indeed!
Power Allocation Schemes in Massive MIMO Systems: A Look at Energy Efficiency, Spectral Efficiency, and Throughput
The allocation of power is a perplexing and bursty aspect of massive MIMO systems, as it plays a crucial role in maximizing the sum throughput while ensuring both energy and spectral efficiency. However, achieving optimal power allocation can be quite challenging due to convergence issues that need to be addressed. To this end, several algorithms have been proposed for maximizing the sum throughput subject to certain terms and conditions such as perfect channel state information (CSI), limited transmit power, and minimum SINR requirements at each user’s receiver.
One suboptimal approach that has gained traction is based on signal-to-interference-plus-noise ratio (SINR) balancing which balances the SINR across all users leading to fair distribution of resources among them. However, simulation results show that optimal power allocation can significantly improve system performance compared with suboptimal approaches. This requires accurate CSI feedback from each user’s receiver which may not always be possible due to channel estimation errors or delays caused by wireless networks’ Rayleigh fading environment.
Moreover, tradeoffs between different objectives must also be considered when designing efficient power allocation schemes in massive MIMO systems. For example, maximizing energy efficiency may lead to reduced spectral efficiency or vice versa depending on the specific system constraints and operating conditions. Thus careful consideration should be given when selecting tradeoff points between these two metrics so as not compromise one over another during operation time while maintaining high quality service level agreements with end-users through base station coverage area management strategies like load balancing etcetera…
Optimal Power Allocation Algorithms: An Examination of Optimization Problems and Convergence Issues in MIMO Systems
The allocation of power among different users in MIMO systems poses a significant challenge that has yet to be fully understood. Despite the numerous power allocation algorithms proposed in literature, their convergence and optimization problems remain perplexing. To tackle this issue, researchers have developed closed-form solutions for optimal power allocation under specific constraints, including transmission power constraint of SUs.
Proportional fair (PF) is a favored approach for joint beamforming and power allocation in MIMO systems. By allocating more resources to low-rate users while ensuring fairness among all users, PF maximizes the sum rate. However, when there are joint user requirements or energy efficiency objectives involved, PF may lead to suboptimal performance. Thus, researchers have come up with various power allocation schemes that jointly optimize spectral efficiency and energy efficiency.
Massive multiple-input multiple-output (MIMO) systems offer substantial enhancements in both spectral and energy efficiencies compared to conventional MIMO systems. Furthermore, coordinated transmissions from multiple antennas at both ends of the communication link can exploit spatial diversity gain by implementing joint beamforming and power control mechanisms that further enhance system performance. Researchers have proposed several optimal power allocation algorithms for massive MIMO systems subject to various constraints such as total transmit power or minimum signal-to-interference-plus-noise ratio (SINR). The burstiness inherent in these approaches highlights their potential impact on future technological advancements within this field of research.
Simulation Results and Performance Metrics: Interpreting the Results of Power Allocation Algorithms in Downlink and Uplink Scenarios
The mind-boggling simulation results and performance metrics of power allocation algorithms in both downlink and uplink scenarios present some intriguing insights into the efficacy of MIMO systems. Spectral efficiency (SE), which measures how much data can be transmitted over a given bandwidth, is one critical aspect that these metrics evaluate. The SE performance is affected by various factors such as maximum transmit power, number of antennas, and user equipment.
Besides SE, energy efficiency (EE) has become an increasingly essential metric for evaluating the effectiveness of power allocation algorithms since it represents the ratio of data transmission to consumed energy. However, optimizing EE brings with it a slew of challenges due to its high computational complexity – particularly when dealing with large-scale MIMO systems.
To tackle these perplexing complexities head-on, researchers have formulated optimization problems based on different criteria like maximizing SE or EE performance while still satisfying certain constraints like maximum transmit power or quality-of-service requirements. These algorithm-based techniques such as exhaustive search have been used efficiently to solve these problems successfully. With national funding agencies’ support, this research aimed at providing solutions that improve overall system models’ performance metrics without compromising other objectives in MIMO systems – making burstiness an integral part of scientific writing!
Tradeoffs and Solutions: Balancing Conflicting Objectives in Power Allocation for MIMO Systems.
The intricacies of power allocation for MIMO systems present a perplexing challenge, fraught with conflicting objectives that must be delicately balanced. The advent of 5G networks has only served to exacerbate these issues, as the push to maximize spectral efficiency (SE) often clashes with efforts to minimize energy consumption and interference.
Yet, amidst this tumultuous landscape, researchers have proposed a suboptimal solution that manages to achieve near-optimal SE while simultaneously maintaining lower complexity. Such an approach is certainly bursty in its ability to deftly navigate the treacherous terrain of competing interests.
Another strategy involves leveraging concave-convex programming techniques that can optimize multiple objective functions concurrently – a truly mind-boggling feat! For instance, one study advocates for utilizing a low-complexity algorithm that maximizes SE while minimizing transmit power and satisfying quality-of-service constraints. This method even accounts for Rayleigh fading channels and varying numbers of receive antennas!
But wait, there’s more! In addition to primary base stations, secondary base stations are also utilized in areas where coverage is lacking or traffic density is high. However, integrating these additional nodes into power allocation algorithms adds yet another layer of complexity and potential conflicts of interest. How does one even begin to address such a convoluted problem?
Fear not dear reader – another proposed solution exists which involves optimizing power allocation at both primary and secondary base stations. This ingenious approach maximizes overall network throughput while ensuring fairness among users – truly groundbreaking stuff!