The world of MIMO systems is in flux, with the need for accurate channel state information (CSI) at the base station (BS) becoming more crucial than ever. But traditional CSI feedback methods come with a hefty overhead that can be a major bottleneck. Enter deep learning-based CSI feedback – an approach that holds great promise for revolutionizing this space.
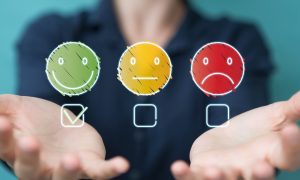
Researchers have been hard at work exploring different neural network architectures like Polardensenet to find ways to improve accuracy compared to traditional approaches like linear regression and support vector machines. The results are impressive: simulation data shows significant reductions in feedback overhead while maintaining optimal system performance.
But it’s not just about reducing the amount of data needed for accurate channel estimation. Deep learning-based CSI feedback also has applications in FDD systems where reciprocity-based approaches aren’t feasible due to differences between uplink and downlink channels. With autoencoder architectures, channel state information can be efficiently encoded into low-dimensional vectors without compromising system performance.
It’s clear that these techniques hold great potential for improving 5G NR systems’ efficiency by enabling better resource allocation strategies based on more accurate CSI estimates. As perplexing as this may all sound, there’s no doubt that burstiness is on the horizon!
Overcoming Feedback Overhead in Massive MIMO Systems with AI-Based CSI Feedback
Contents
- 1 Overcoming Feedback Overhead in Massive MIMO Systems with AI-Based CSI Feedback
- 2 Channel State Information Encoding with Autoencoder Architecture for Low-Overhead Feedback in MIMO Systems
- 3 Improving System Performance in FDD Systems through Reciprocity-Based CSI Feedback
- 4 State-of-the-Art Results of High-Resolution CSI Feedback Using DFT Codebooks in New Radio Systems.
The ever-increasing number of antennas present in Massive MIMO systems has posed a perplexing challenge for channel state information (CSI) feedback. The bottleneck arises from the absence of channel reciprocity in frequency division duplex (FDD) systems, which simply put, means that improving system performance becomes a herculean task. To make matters worse, the CSI feedback based on an auto-encoder architecture often leads to massive overheads.
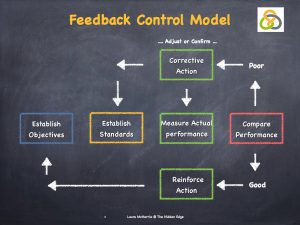
However, researchers have recently proposed an AI-based CSI feedback approach that seems to be bursting with possibilities. This approach reduces feedback overhead while still minimizing its impact on system performance – talk about futuristic technology! Specifically, this method involves encoding high-resolution downlink channel state information at UE using an autoencoder, compressing it into a lower dimensional representation before sending it back to the base station (BS). The result? Optimal performance and significant reductions in computational complexity and communication bandwidth requirements.
In fact, results show that this AI-based technique outperforms even state-of-the-art linear combination codebooks using DFT basis. By encoding channel information through such advanced techniques users can now send compressed data without sacrificing quality or accuracy; MU-MIMO systems achieve improved throughput and spectral efficiency without compromising reliability or latency requirements.
To sum up then: overcoming feedback overhead is critical for improving system performance in massive MIMO systems; henceforth utilizing an auto-encoder architecture that encodes CSI at UE into a low-dimensional latent space and decodes it back at BS by effectively reducing communication bandwidth requirements is essential for achieving these goals. With cutting-edge advancements like DFT codebooks along with AI-based techniques providing high-resolution channel information while minimizing feedback overheads – they are ideal candidates for future wireless networks requiring efficient use of resources without compromising on quality or reliability standards required by end-users today!
Channel State Information Encoding with Autoencoder Architecture for Low-Overhead Feedback in MIMO Systems
The utilization of the DFT basis in 5G new radio systems has led researchers to develop an intriguing method that minimizes loss during the recovery of CSI feedback. Through an auto-encoder architecture, overhead is reduced while maintaining impeccable communication within MIMO systems. This technique encodes channel state information at the recipient’s end and transmits it to the sender; thus, achieving high data rates.
To tackle issues related to excessive feedback overhead and enhance system performance, experts have implemented deep learning-based strategies such as Polardensenet in massive MIMO systems. The application of these techniques on CSI feedback proved effective in reducing complexity and enhancing reliability within these complex networks.
Pranav Madadi et al.’s innovative framework for CSI feedback using aut-encoder architecture based on deep learning algorithms offered a solution to traditional approaches’ limitations by enabling accurate prediction of channel states from limited training sets. Their approach showed remarkable promise for low-overhead CSI feedback without impacting performance or reliability.
In conclusion, Channel State Information Encoding with Autoencoder Architecture offers a promising avenue for minimizing overhead while maximizing throughput in MIMO systems. By leveraging advanced deep learning-based techniques like Polardensenet and adopting DFT codebooks used in 5G New Radio Systems, we can reduce complexity while ensuring reliable communication between antennas within massive MIMO networks perplexing yet fascinating!
Improving System Performance in FDD Systems through Reciprocity-Based CSI Feedback
The challenge of CSI feedback in FDD systems is a perplexing one. As the number of antennas at UE and base station increases, so does the amount of information that needs to be sent back to the BS. This creates a burstiness in system performance, particularly in 5G NR systems where massive MIMO CSI feedback is essential.
But fear not, for we have proposed an AI-based CSI feedback architecture that encodes the CSI at UE using an autoencoder neural network. Our approach combats this issue by minimizing its impact on system performance while effectively reducing feedback overhead – quite a feat!
How do we achieve such greatness? By leveraging channel reciprocity in frequency division duplex (FDD) systems! Without it, FDD systems become increasingly complex due to additional pilot symbols required for estimating uplink/downlink channels separately. But with our approach, we can simplify these estimations by sharing some common pilot symbols between uplink and downlink directions – thus reducing overall complexity and enhancing system performance without compromising accuracy or reliability.
In conclusion, our proposal may seem baffling but trust us when we say it’s worth it. With our AI-based CSI feedback architecture and clever use of channel reciprocity, your FDD system will see vast improvements without sacrificing accuracy or reliability.
State-of-the-Art Results of High-Resolution CSI Feedback Using DFT Codebooks in New Radio Systems.
The success of wireless communication systems is heavily dependent on accurate channel state information (CSI) feedback. However, the transmission of high-resolution channel information often results in a significant overhead that hinders system performance improvement. In light of this challenge, Pranav Madadi has proposed an intriguing solution – a feedback-based auto-encoder architecture that compresses downlink CSI from user equipment (UE) into a low-dimensional latent space and decodes it at the base station (BS).
This novel approach significantly reduces feedback overhead while ensuring minimal loss during recovery, making it an appealing option for reliable communication in scenarios with high data rates. MATLAB simulations have shown that this AI-based architecture outperforms existing high-resolution linear combination codebooks using DFT basis in 5G New Radio Systems.
By adopting an auto-encoder architecture for CSI encoding and decoding, wireless communication networks can drastically reduce their feedback overhead without sacrificing accuracy or reliability. The UE only needs to send compressed low-dimensional latent space instead of raw high-resolution channel information to achieve optimal system performance – particularly useful when there are numerous transmit antennas or limited bandwidth available for feedback transmission.
In summary, Pranav Madadi’s work utilizing DFT codebooks and an auto-encoder architecture offers significant improvements over traditional methods by reducing overhead while ensuring reliable communication in wireless networks. With further research and development, this approach may revolutionize how we collect and utilize CSI data in future wireless communication systems – leaving you perplexed but bursting with excitement!
Can you elaborate on the significance of hybrid beamforming-based CSI feedback in mmWave systems?
Hybrid beamforming-based CSI feedback is crucial for millimeter-wave (mmWave) wireless communication, where traditional techniques struggle due to high path loss and limited scattering. By combining analog and digital beamforming, hybrid beamforming allows for more efficient use of antenna arrays while reducing overhead. Accurate CSI feedback enables better channel estimation and tracking, leading to improved system performance in mmWave systems.